The Future of Business: Unlocking Potential with Data Labeling Machine Learning
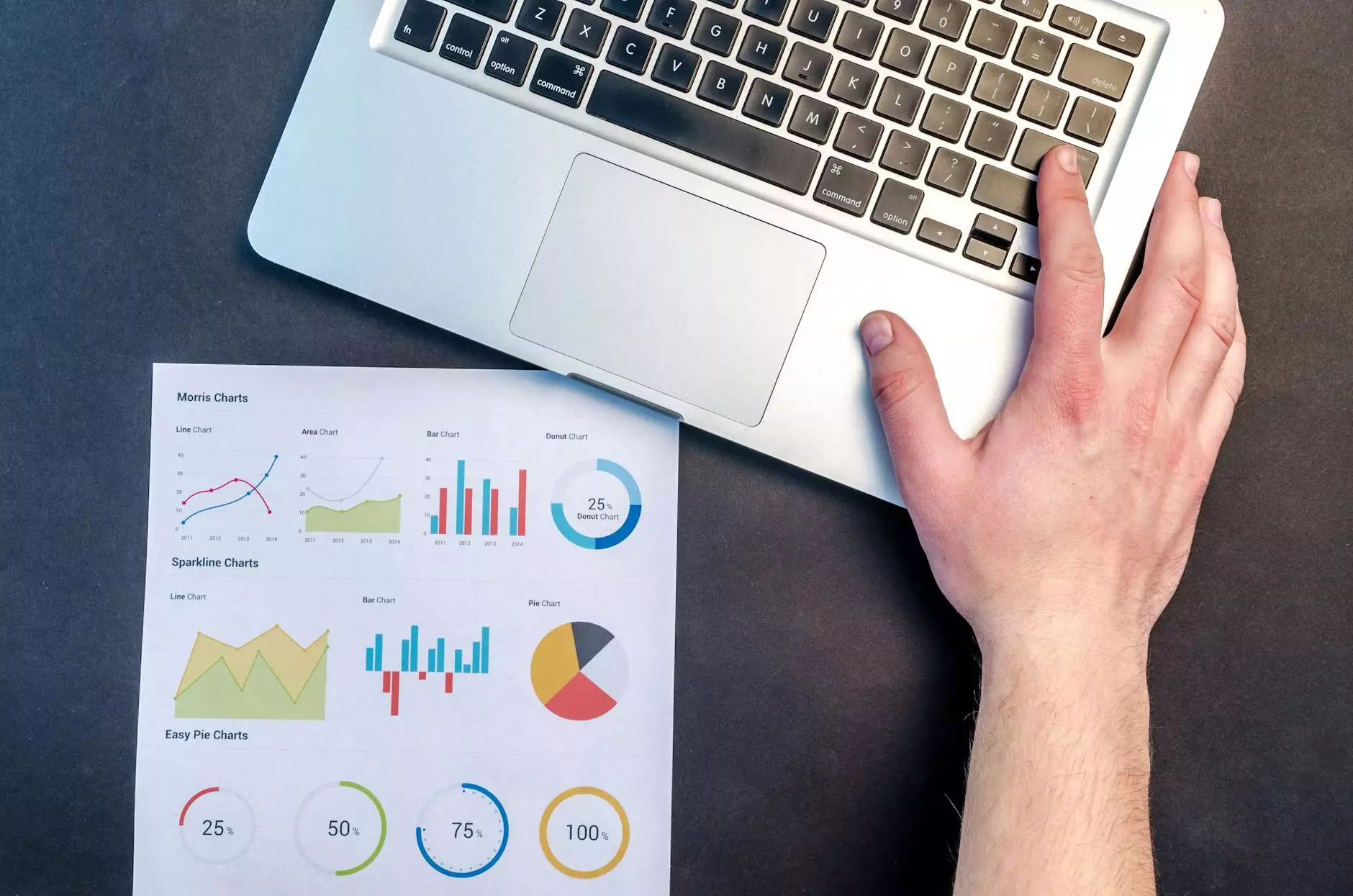
In today's rapidly evolving digital landscape, businesses are increasingly leaning on advanced technologies to gain a competitive edge. One of the most crucial components of the tech ecosystem is data labeling machine learning, which serves as a cornerstone for artificial intelligence (AI) and machine learning (ML) models. By understanding the intricacies of this technology, businesses can enhance their operational efficiency, improve customer experiences, and ultimately, drive growth.
Understanding Data Labeling in Machine Learning
Data labeling is the process of annotating data to make it suitable for supervised machine learning algorithms. Without labeled data, machine learning models cannot learn effectively, as they rely on pre-defined labels to identify patterns and make predictions.
Types of Data Labeling
- Image Annotation: Involves labeling images for object detection, image segmentation, and image classification.
- Text Annotation: Includes tasks like entity recognition, sentiment analysis, and intent classification.
- Audio Annotation: Used for speech recognition, sound classification, and speaker identification.
Why Data Labeling is Vital for Businesses
As machine learning models need extensive and accurately labeled datasets to function optimally, the importance of effective data labeling cannot be overstated. Here are several reasons why businesses need to invest in data labeling:
1. Improved Model Accuracy
High-quality labeled data ensures that ML models can recognize and interpret data correctly, leading to higher accuracy. Data labeling machine learning enables businesses to train models that can outperform their competitors in predictive capabilities.
2. Faster Time to Market
Automating the labeling process through advanced platforms can significantly speed up the time to market. This agility allows businesses to adapt quickly to changing market conditions and customer demands.
3. Enhanced Decision Making
With better data labeling, businesses can derive actionable insights from data analytics, leading to informed decision-making and strategic planning.
Choosing the Right Data Annotation Tool and Platform
When selecting a data annotation tool or platform, businesses should consider several factors to ensure they meet their specific needs:
Cost Effectiveness
Budget constraints are a reality for many businesses. Evaluating the cost-effectiveness of different tools and platforms can help reduce overall expenditure without compromising quality.
Scalability
As the volume of data grows, businesses should opt for a solution that can scale efficiently. A robust data annotation platform must handle increasing amounts of data without a hitch.
Integration Capabilities
Choose tools that seamlessly integrate with existing workflows and systems. This integration minimizes disruption and allows for smoother operations.
User-Friendliness
A user-friendly interface empowers teams to adopt the tool more quickly, resulting in less training time and faster deployment.
Keylabs.ai: Leading the Way in Data Annotation
At Keylabs.ai, we provide a state-of-the-art data annotation platform designed to cater to the diverse needs of businesses. Our platform supports various data types, including images, text, and audio, making it a versatile solution for any industry. Here’s how Keylabs.ai stands out:
Comprehensive Annotation Solutions
We offer a wide range of annotation solutions, from simple image tagging to complex video segmentation, ensuring that every aspect of your data is covered.
AI-Powered Automation
Our platform leverages AI to automatically suggest labels, significantly reducing the time and effort required for data annotation while maintaining high accuracy.
Quality Assurance and Control
With built-in quality assurance processes, we ensure that all labeled data meets the highest standards, so your machine learning models can learn effectively.
The Role of Data Labeling Machine Learning in Different Industries
Data labeling is not confined to a single industry; its applications are widespread across various sectors. Let's explore how it is transforming industries:
1. Healthcare
In healthcare, labeled data is utilized for diagnosing diseases from medical images and predicting patient outcomes. Enhanced data labeling leads to better AI models capable of assisting healthcare professionals with diagnostic support.
2. Automotive
In the autonomous vehicle sector, data labeling is critical for training models to recognize road signs, pedestrians, and other vehicles, thereby ensuring safety in automated driving systems.
3. Retail
Retail businesses leverage data labeling for customer sentiment analysis, inventory management, and personalized marketing strategies. By understanding customer behavior patterns, retailers can tailor their offerings to better meet consumer needs.
4. Finance
In finance, labeled data helps in fraud detection, risk assessment, and algorithmic trading. Effective data labeling can significantly reduce financial risk and enhance profitability.
Challenges in Data Labeling
While data labeling provides immense benefits, it also comes with challenges. Understanding these challenges can help businesses strategize better:
Quality Control
Ensuring high-quality labeled data is vital, as inaccuracies can lead to poor model performance. Establishing quality control measures is essential to mitigate this risk.
Time Consumption
The data labeling process can be time-consuming, especially when dealing with large datasets. Leveraging automated tools can alleviate this concern.
Staff Training
Staff must be adequately trained to use data labeling tools effectively. Continuous learning and skill development are necessary for maximizing the potential of data labeling platforms.
Future Trends in Data Labeling Machine Learning
The future of data labeling in machine learning is bright, with several emerging trends poised to shape its evolution:
1. Increased Automation
As AI technology advances, we will see a surge in automated data labeling solutions that can handle complex tasks with minimal human intervention.
2. Enhanced Collaboration Tools
Future platforms will likely focus on facilitating collaboration among teams to streamline the data labeling process and improve outcomes.
3. Real-Time Labeling
Innovations may lead to real-time data labeling, allowing businesses to label data as it comes in, thus keeping up with the fast-paced digital world.
Conclusion: Embrace the Future with Data Labeling
The integration of data labeling machine learning into business practices is not just a trend; it is a fundamental requirement for staying competitive in the digital age. By investing in high-quality data annotation tools and platforms like Keylabs.ai, businesses can equip themselves with the necessary tools to thrive.
In this ever-evolving landscape, those who prioritize accurate data labeling will undoubtedly enjoy enhanced operational success, better customer insights, and sustainable growth. The future is bright for businesses that embrace these technologies—are you ready to take the leap?